CCIM Connections
The Official Publication of The CCIM Institute
Image
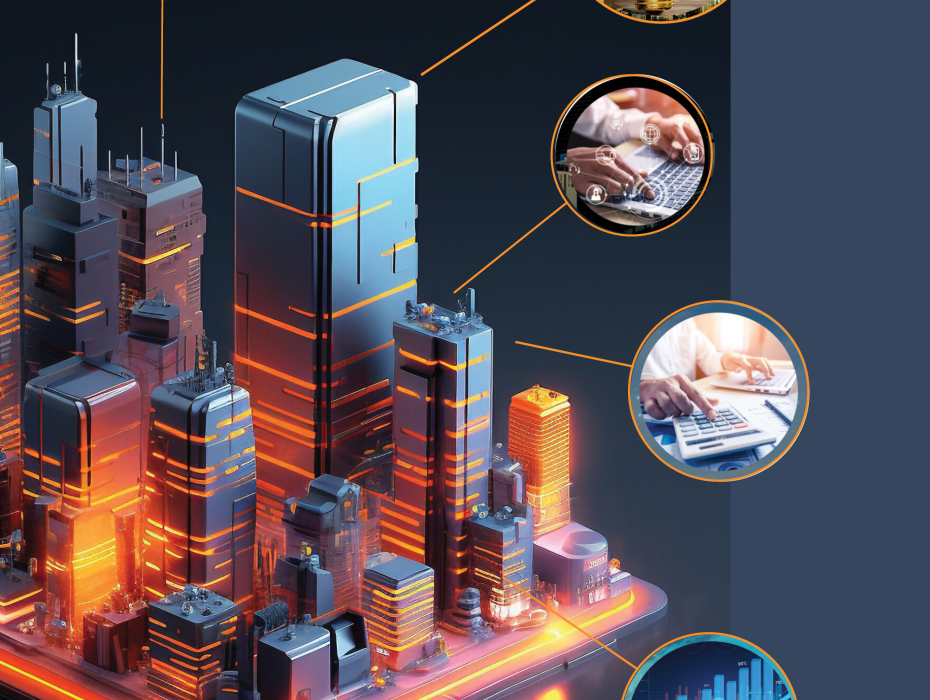
Image
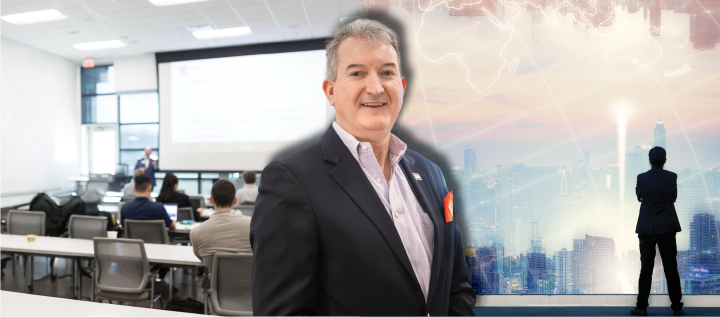
Spring 2025 Connections
From Student to Leader
Steve Rich’s Journey to Global President and his Vision for The CCIM Institute
Read Article
The Latest Trending Articles
Featured Articles by Issue
Stay Up to Date on All Issues
Hero image
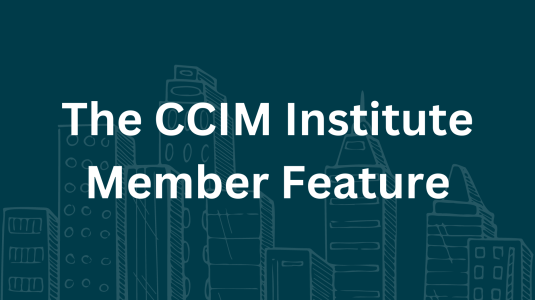
Intro Text
FROM ENVIRONMENTAL SCIENTIST TO LAND SALES, GONZALEZ FOUND A SECOND CAREER IN COMMERCIAL REAL ESTATE.
Intro Text
CCIM Designees are traversing the world to make deals happen.
Hero image
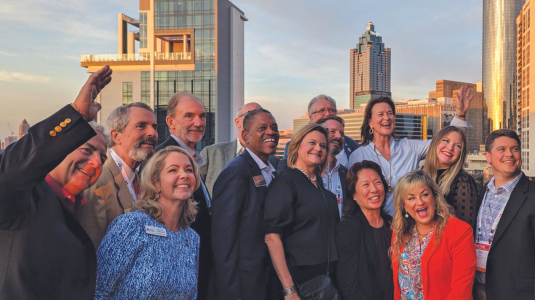
Intro Text
Did we get to see you in-person in 2023?
Intro Text
A glimpse into the experiences of two out of the 166 new Designees who were pinned in April 2024.